Note
This Python sample may be slow depending on the event rate of the scene and the configuration of the algorithm. We provide it to allow quick prototyping. For better performance, look at the corresponding C++ sample.
Spatter Tracking using Python
The Python bindings of Metavision SDK Analytics provide the metavision_sdk_analytics.SpatterTrackerAlgorithm
class for spatter tracking or tracking simple non-colliding moving objects. This tracking algorithm is a lighter
implementation of metavision_sdk_analytics.TrackingAlgorithm
, and it suits only to non-colliding objects.
The sample metavision_spatter_tracking.py
shows how to use
metavision_sdk_analytics.SpatterTrackerAlgorithm
to track objects.
The source code of this sample can be found in
<install-prefix>/share/metavision/sdk/analytics/python_samples/metavision_spatter_tracking
when installing Metavision SDK from installer or packages. For other deployment methods, check the page
Path of Samples.
Expected Output
Metavision Spatter Tracking sample visualizes all events and the tracked objects by showing a bounding box around each tracked object with an ID of the tracked object shown next to the bounding box:
Setup & requirements
By default, Metavision Spatter Tracking looks for objects of size between 10x10 an 300x300 pixels.
Use the command line options --min-size
and --max-size
to adapt the sample to your scene.
How to start
To start the sample based on the live stream from your camera, run:
python metavision_spatter_tracking.py
To start the sample based on recorded data, provide the full path to a RAW file (here, we use a file from our Sample Recordings):
python metavision_spatter_tracking.py -i sparklers.raw
In sparklers.raw from Sample Recordings, the speed of sparkles is very high and the sparkles are very tiny. To be able to track the sparkles in this particular file, the tracking parameters should be tuned, for example:
python metavision_spatter_tracking.py -i sparklers.raw --accumulation-time-us 50 --cell-width 4 --cell-height 4 --activation-ths 2
To check for additional options:
python metavision_spatter_tracking.py -h
Algorithm Overview
Spatter Tracking Algorithm
The tracking algorithm consumes CD events and produces tracking results (i.e.
metavision_sdk_analytics.EventSpatterClusterBuffer
).
Those tracking results contain the bounding boxes with unique IDs of tracked objects.
The algorithm is implemented in an asynchronous way and processes time slices of fixed duration. This means that, depending on the duration of the input time slices of events, the algorithms might produce 0, 1 or N buffer(s) of tracking results.
Like with any other asynchronous algorithm, we need to specify the callback that will be called to retrieve the tracking results when a time slice has been processed:
ents iterator on Camera or event file
terator = EventsIterator(input_path=args.event_file_path, start_ts=args.process_from,
max_duration=args.process_to - args.process_from if args.process_to else None,
delta_t=args.accumulation_time_us)
rgs.replay_factor > 0 and not is_live_camera(args.event_file_path):
mv_iterator = LiveReplayEventsIterator(mv_iterator, replay_factor=args.replay_factor)
The tracking algorithm consists mainly of two parts. First, the input events are continuously accumulated in a user-defined grid during
the time slice. Then, at the end of the time slice, cells where the event count is high are marked as activated. Clusters are formed from connected active cells and a bounding box is defined around each of them.
Small and big clusters are filtered out depending on the user’s input for their desired size. Finally the newly detected clusters are matched with previously detected clusters, based on their center-to-center distance,
to update the position of the tracked Metavision::EventSpatterCluster
.
The algorithm’s steps are illustrated below:
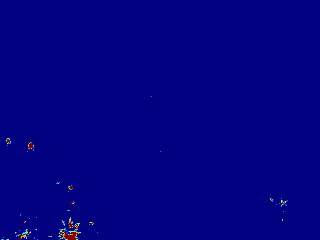
Heatmap of events count during a time slice
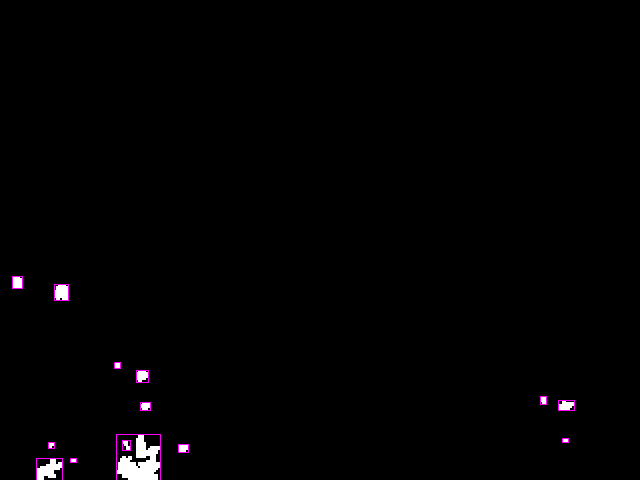
Bounding box around detected clusters
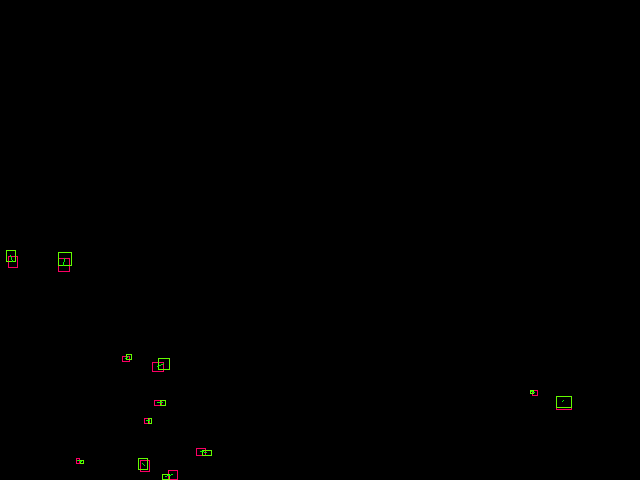
Matching previous clusters (in red) to new detected ones (in green)
Code Overview
Pipeline
The sample implements the following pipeline:

Note
The pipeline also allows to apply filters on the events using the algorithms
SpatioTemporalContrastAlgorithm
that can be enabled and configured using the --stc_threshold
command line option.
Other software filters could be used like ActivityNoiseFilterAlgorithm
or TrailFilterAlgorithm
.
Alternatively, to filter out events, you could enable some of the ESP blocks of your sensors.
Slicer initialization
The metavision_core.event_io.EventsIterator
is used to produce events slices with the appropriate duration:
# Events iterator on Camera or event file
mv_iterator = EventsIterator(input_path=args.event_file_path, start_ts=args.process_from,
max_duration=args.process_to - args.process_from if args.process_to else None,
delta_t=args.accumulation_time_us)
if args.replay_factor > 0 and not is_live_camera(args.event_file_path):
mv_iterator = LiveReplayEventsIterator(mv_iterator, replay_factor=args.replay_factor)
Events of the slice are directly processed on the fly in the iterator’s loop.
Processing
In the iterator’s loop:
input events are optionally filtered and then passed to the frame generation and Spatter Tracking algorithms
we retrieve the results of the Spatter Tracking algorithm
generate an image where the bounding boxes and the IDs of the tracked objects are displayed on top of the events
# Process events
filtered_evs = SpatioTemporalContrastAlgorithm.get_empty_output_buffer() if do_filtering else None
for evs in mv_iterator:
ts = mv_iterator.get_current_time()
# Dispatch system events to the window
EventLoop.poll_and_dispatch()
# Process events
if do_filtering:
stc_filter.process_events(evs, filtered_evs)
buffer = filtered_evs if do_filtering else evs
events_frame_gen_algo.process_events(buffer)
spatter_tracker.process_events(buffer, ts, clusters)
clusters_np = clusters.numpy()
for cluster in clusters_np:
log.append([ts, cluster['id'], int(cluster['x']), int(cluster['y']), int(cluster['width']),
int(cluster['height'])])
events_frame_gen_algo.generate(ts, output_img)
tracked_clusters.update_trajectories(ts, clusters)
draw_tracking_results(ts, clusters, output_img)
draw_no_track_zones(output_img)
tracked_clusters.draw(output_img)
window.show_async(output_img)
if args.out_video:
video_writer.write(output_img)
if window.should_close():
break
Display
Finally, the generated frame is displayed on the screen, the following image shows an example of output:
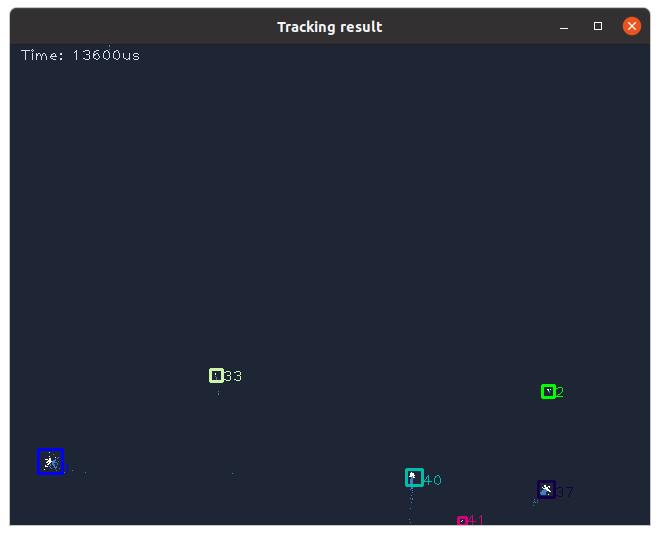
Going further
Estimate Speed of a tracked object
The sample uses the class metavision_sdk_analytics.EventSpatterClusterBuffer
(the python binding of the C++ structure Metavision::EventSpatterCluster
) which produces tracking results
containing the bounding boxes with unique IDs of tracked objects. The coordinates of bounding boxes and the current
track’s timestamp are also given by this structure.
Hence, for two different positions of a tracked object, it should be possible to estimate its speed for a given time slice.