ArUco Marker Tracking using C++
The CV3D API provides algorithms to track ArUco markers in 3D. It operates on 2D data from Prophesee event-based camera.
The sample metavision_aruco_marker_tracking.cpp
shows how to implement a pipeline for detecting and tracking an
ArUco marker in 3D.
This sample shows a specific utilisation of Metavision::Model3dTrackingAlgorithm
to track ArUco Marker.
The source code of this sample can be found in <install-prefix>/share/metavision/sdk/cv3d/cpp_samples/metavision_aruco_marker_tracking
when installing Metavision SDK from installer or packages. For other deployment methods, check the page
Path of Samples.
Expected Output
This sample outputs the pose of the camera with respect to the marker.
How to start
To run this sample, you will need to provide an event file (RAW, DAT or HDF5) containing a sequence in which the ArUco marker to be tracked appears (offline mode) and a JSON file containing the calibration of the camera used for the record (or the camera used in live mode). You can find an archive containing examples of such files in our Sample Recordings.
Optionally, you can also provide a camera settings file to tune the biases when the sample is executed in live mode.
To run the sample, first, compile the sample as described in this tutorial.
Then, to start the sample using a RAW file, run:
Linux
./metavision_aruco_marker_tracking -i path/to/object.raw -c path/to/calibration.json
Windows
metavision_aruco_marker_tracking.exe -i <path>/object.raw -c <path>/calibration.json
If you want to start the sample based on the live stream from your camera, you will need to provide a
camera settings file with bias values wisely chosen for this specific application.
To do so, you can use the command line option --input-camera-config
(or -j
) with a JSON file containing the settings.
To create such a JSON file, check the camera settings section.
Here is how to launch the sample with a JSON camera settings file:
Linux
./metavision_aruco_marker_tracking -c path/to/calibration.json -j path/to/my_settings.json
Windows
metavision_aruco_marker_tracking.exe -c path\to\calibration.json -j path\to\my_settings.json
To check for additional options:
Linux
./metavision_aruco_marker_tracking -h
Windows
metavision_aruco_marker_tracking.exe -h
Note
If tracking gets lost sometimes, you can try to adjust those tracking parameters:
--n-events N_EVENTS
: number of events after which a tracking step is attempted. (default: 5000)
--n-us N_US
: amount of time after which a tracking step is attempted. (default: 10000)
Note that smaller n-events
or n-us
makes algorithm more robust to higher speed motion but slower (latency increases)
as well. So to make it faster, make sure your sensor biases are tuned correctly for your application.
You should try to reduce noise as much as possible. Indeed not only noise makes the algorithm slower, but it also makes it less robust. Depending on the speed of your objects (especially for high-speed objects), you might have to tune the sensor biases to get better data. Using some filtering (see activity filter or trail filter) and/or Region of Interest (ROI) may help to speed up the detection algorithm and reduce the latency.
Code Overview
Processing Pipeline
The Metavision::ArucoMarkerDetectionAlgorithm
can be instantiated with a reference to a Metavision::CameraGeometryBase
instance and the size of the marker in meters.
Then the Metavision::Model3dTrackingAlgorithm
can be instantiated with:
a reference to a
Metavision::CameraGeometryBase
instancea reference to a
Metavision::Model3d
instancea reference to a
Metavision::MostRecentTimestampBufferT
instance that it will fill with the input events buffers
detection_algo_ = std::make_unique<Metavision::ArucoMarkerDetectionAlgorithm>(*cam_geometry_, corners_list,
edges_list, config.marker_size);
tracking_algo_ = std::make_unique<Metavision::Model3dTrackingAlgorithm>(*cam_geometry_, model_, time_surface_,
config.tracking_params_);
This sample implements the following basic state machine:
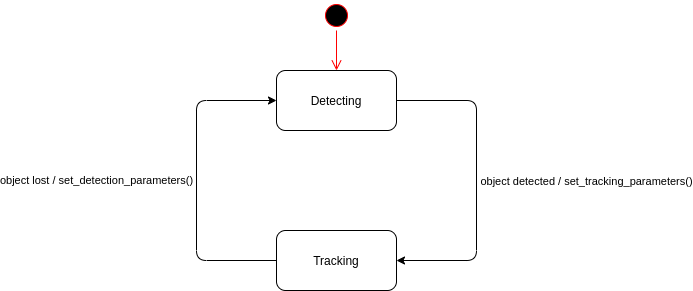
Both algorithms process time slices of events and output a camera’s pose in case of success. Those time slices can be created according to different strategies (i.e. fixed number of events, fixed duration or a mixed condition).
The Metavision::Model3dTrackingAlgorithm
is meant to track an object while the user is moving very fast. To
track that object during fast motions the algorithm relies on the camera’s high temporal resolution which makes the
object very close from its previous location from one step to another. This hypothesis enables the implementation of
very efficient methods (that still remain valid for slow motions). As a consequence, to work correctly, this
algorithm has to be called at a high frequency when the user is moving fast but can be called at a very low frequency
when the user is moving slowly. One way to get this behavior is to feed the algorithm with time slices having a fixed
number of events. By doing so, the output camera poses frequency automatically adapts to the camera’s speed.
In contrast to the Metavision::Model3dTrackingAlgorithm
, the
Metavision::ArucoMarkerDetectionAlgorithm
algorithm is meant to detect the marker after an integration time.
The algorithm can be called at a relative low frequency. As a result, big fixed duration time slices (e.g. 10ms) are well
suited for this algorithm.
To address this, the algorithms could have been implemented in an asynchronous way, each producing its result (i.e. calling the asynchronous output callback) according to its configuration (i.e. N events, N us or mixed), but this would have made the synchronization between them complicated during state changes.
Instead, the problem is solved upstreams by putting a Metavision::SharedEventsBufferProducerAlgorithm
between the camera and the algorithms and whose configuration can be changed at runtime. Here is the pipeline
implemented in the sample:
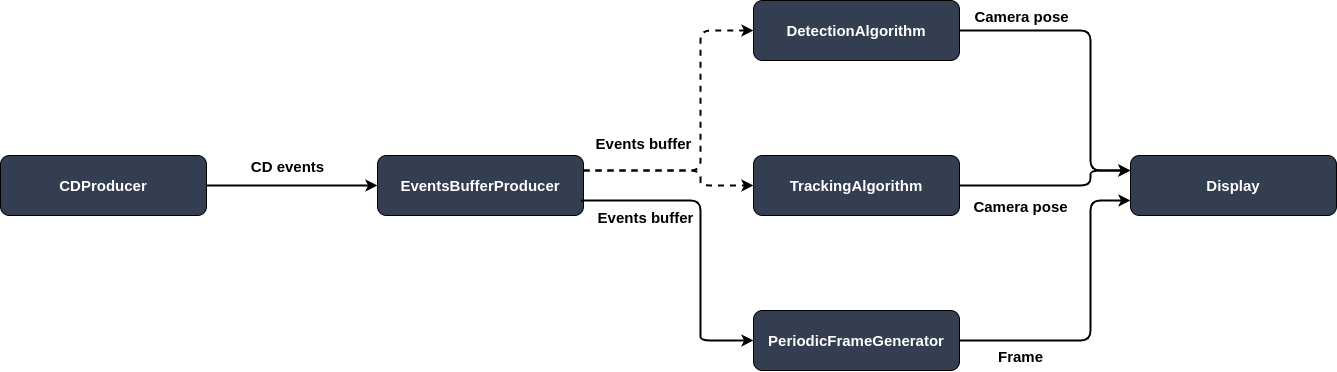
For technical reasons, further detailed in the code, the produced buffers cannot directly be processed in the
Metavision::SharedEventsBufferProducerAlgorithm
’s output callback. As a consequence, when the output
callback is called, the produced buffer is pushed into a queue so that it can be processed later:
Metavision::SharedEventsBufferProducerParameters params;
auto cb = [&](Metavision::timestamp ts, const Buffer &b) {
if (!b->empty())
buffers_.emplace(b);
};
producer_ = std::make_unique<Metavision::SharedCdEventsBufferProducerAlgorithm>(params, cb);
In the camera’s callback, events are first processed by the producer and then the time slices, that may have been
produced meanwhile, are processed by the Metavision::ArucoMarkerDetectionAlgorithm
and
Metavision::Model3dTrackingAlgorithm
.
void cd_processing_callback(const Metavision::EventCD *begin, const Metavision::EventCD *end) {
producer_->process_events(begin, end);
process_buffers_queue();
}
And the full buffers queue processing loop:
while (!buffers_.empty()) {
const bool prev_is_tracking = is_tracking_;
const auto buffer = buffers_.front();
buffers_.pop();
const auto begin = buffer->cbegin();
const auto end = buffer->cend();
bool enabled_tracking = false;
if (is_tracking_) {
is_tracking_ = tracking_algo_->process_events(begin, end, T_c_w_);
} else {
if (detection_algo_->process_events(begin, end, detected_markers_)) {
// tries to find the marker to track
// if found, updates the model and the camera pose
if (process_detected_markers()) {
// we wait for several consecutive detections before considering the model as detected to avoid
// false positive detections
enabled_tracking = (++n_detection_ >= config_.n_detections_);
}
}
// filling time surface manually since the tracking will use it
for (auto it = begin; it != end; ++it) {
time_surface_.at(it->y, it->x, it->p) = it->t;
}
}
// the frame generation algorithm processing can trigger a call to show_async which can trigger a reset of
// the tracking if the space bar has been pressed.
frame_generation_algo_->process_events(begin, end);
// we want to update the tracking state AFTER the frame_generation_algo_ produced the frames. Indeed, after
// the successful detection of the marker, its pose corresponds to its last pose observed during the batch
// of events, while several frames might be produced during this batch. However, we don't want to display
// the marker on intermediate frames where it wouldn't match the events drawn.
if (enabled_tracking) {
is_tracking_ = true;
frame_generation_algo_->force_generate();
}
if (prev_is_tracking != is_tracking_) {
if (is_tracking_)
set_tracking_params(std::prev(buffer->cend())->t); // triggers the creation of a new buffer
else
set_detection_params(); // triggers the creation of a new buffer
}
}
Note
We can note from the code that we do not switch to the tracking state as soon as the object is detected. Instead, we wait for
N
detections to reduce false positive ones. Since ArUco detection is quite robust,N
could be set to 1 or 2.The detection algorithm does not use the time surface, so we need to fill it manually in the processing loop before switching to the tracking configuration
Note
In the sample, the tracking can also be reset by pressing the space bar key
Detection Algorithm
The detection algorithm uses ArUco Nano, a condensed library to detect markers of dictionary
ARUCO_MIP_36h12
. Make sure your application uses one of the markers of this dictionary
or else the detection won’t succeed.
See also
For more informations about ArUco detection, please refer to:
“Speeded up detection of squared fiducial markers”, Francisco J.Romero-Ramirez, Rafael Muñoz-Salinas, Rafael Medina-Carnicer, Image and Vision Computing, vol 76, pages 38-47, year 2018
“Generation of fiducial marker dictionaries using mixed integer linear programming”,S. Garrido-Jurado, R. Muñoz Salinas, F.J. Madrid-Cuevas, R. Medina-Carnicer, Pattern Recognition:51, 481-491,2016
The detection algorithm processes time slices of events and integrate them into a binary image that can be read by ArUco Nano. We use the provided function to estimate the pose of the camera in relation to the marker.
Note
ArUco Nano can potentially detect and estimate the pose of several markers present in the camera frame. You can decide to track
a specific marker by specifying its ID with the --id-to-track
parameter. By default, we track the first marker detected.
Utils functions are provided to output the detected marker and the computed camera pose to JSON files. Those files could be used as inputs for the Model3d Tracking C++ Sample.
Tracking Algorithm
The tracking algorithm proceeds as follows:
visible edges of the model (here a marker) are recovered,
points are sampled along each edge, called support points,
matches for each support point are search in the timesurface,
the associations of points and matches are used in a Gauss-Newton optimization to compute the pose of the camera in relation to the model.
Matches are determined by searching along the normal of the edge for the pixel with the latest timestamp. Point-match associations are weighted in relation to the most recent timestamp in the lot. Default weights for the most recent and oldest timestamp can be changed in the pipeline parameters.
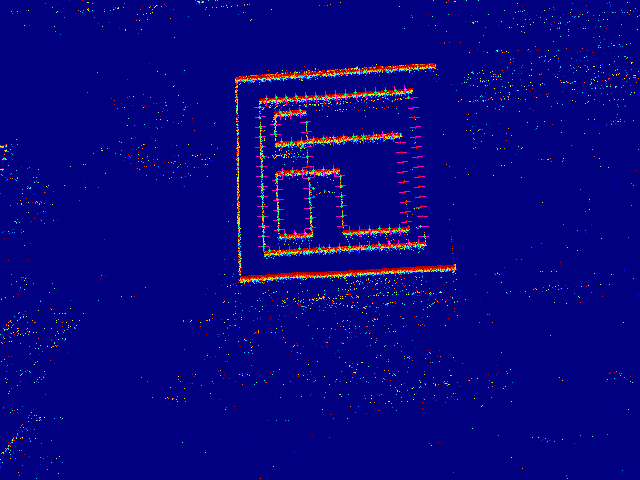
Tracking step during an overall vertical movement
The timesurface is pseudo-colored from most-recent timestamp (red) to oldest allowed timestamp (dark blue). A pink line is drawn for each support point, representing the area of search. Green points denote good matches and red points denote no match.
Note
If you experience latency in your tracking you can try to reduce the parameter --tracking-search-radius
to limit the search for matches to closer pixels, or increase the parameter
--tracking-support-point-step
to increase the inter-distance between support points, and therefore reduce their overall number. That could however make the tracking less robust.
Reciprocally, if the tracking gets lost, you can try to increase the parameter --tracking-search-radius
to expand the search for matches to more pixels, and/or reduce
the parameter --n-events
so that less events are processed between tracking steps and so the marker would have moved a lesser distance. That could however increase
the latency of the overall pipeline.
Display
For the display we use the Metavision::PeriodicFrameGenerationAlgorithm
asynchronous algorithm which
generates frames from events at a fixed frame rate. When a new frame is available we draw the model’s edges on top of it
using the last known camera’s pose. When the pipeline is in the detection state, tracked and non tracked edges are
drawn using different colors:
void frame_callback(Metavision::timestamp ts, cv::Mat &frame) {
cv::putText(frame, std::to_string(ts), cv::Point(0, 10), cv::FONT_HERSHEY_DUPLEX, 0.5,
(is_tracking_ ? cv::Scalar(0, 255, 0) : cv::Scalar(0, 0, 255)));
// Be careful, here the events and the 3D model are not rendered in a tightly synchronized way, meaning that
// some shifts might occur. However, most of the time they should not be noticeable
if (is_tracking_) {
Metavision::select_visible_edges(T_c_w_, model_, visible_edges_);
Metavision::draw_edges(*cam_geometry_, T_c_w_, model_, visible_edges_, frame, cv::Scalar(0, 255, 0));
cv::putText(frame, "tracking", cv::Point(0, 30), cv::FONT_HERSHEY_DUPLEX, 0.5, cv::Scalar(0, 255, 0));
} else {
cv::putText(frame, "detecting", cv::Point(0, 30), cv::FONT_HERSHEY_DUPLEX, 0.5, cv::Scalar(0, 0, 255));
}
if (video_out_)
video_out_->write(frame);
if (window_)
window_->show_async(frame);
}
Warning
As pointed out in the code, the background frame and the 3D model’s edges may not be perfectly aligned. However this should be imperceptible in practice.